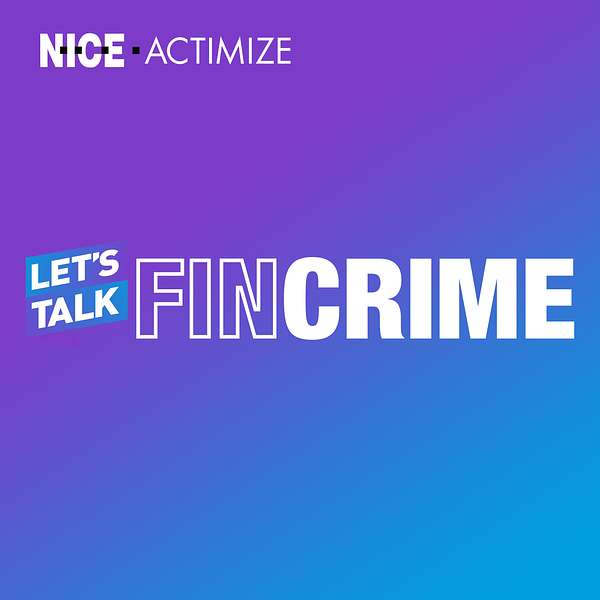
Let's Talk FinCrime
Let's Talk FinCrime
Episode 15: Let’s Talk FinCrime with AI & Machine Learning
What is AI and machine learning and how are they being used in the fight against financial crime?
In this episode of Let’s Talk FinCrime, hear from Parit Patel, NICE Actimize Director of Solutions Consulting, as he discusses changes in managing financial crime risk brought on by AI and machine learning, as well as its general growth outlook over the coming years.
Parit Patel is the Solutions Director for EMEA and APAC at NICE Actimize. Parit has been part of this team for over two years and focuses on helping customers design and develop a financial crime roadmap that makes them more accurate and effective. Before joining NICE Actimize, Parit spent nearly 20 years across a number of technology start-ups - specifically within organizations that focused on AI and machine learning.
You can hear more of our conversation with Parit by visiting actimize.nice.com/podcast
Welcome to Let's talk in crime, the show where we explore the human side of financial crime. We cover not only the big industry trends, but how you can truly protect yourself and your assets. I'm 10,000, just one of the hosts for season three. I'm a subject matter expert within the AMA line of business at nights active mice base in our New York office. Prior to that, I was with a larger financial institution out of Chicago for 10 years, implementing AML solutions and processes. For season three, we're doing something a little bit different. Over the next six episodes, you'll hear from a few voices from around nice atomize as we have conversations with some of the most interesting people in the financial world. In today's episode, I'm joined by parrot Patel, also known as active PR, is the head of a solution consulting firm. Welcome, and can you give me a little bit more information about yourself? Sure, thanks, Ted. So like Ted said, my name is parrot, I run the solutions team for atomise. In an era back, and I've got a very different background from Ted, I actually don't have much of a fin crime background, I've been active for about three years. And before then, I spent the last 20 odd years in startups and startups that really focused on AI. In particular, I like to pretend I'm a bit of a hipster, and was doing AI machine learning before it became cool again. It's really interesting, bringing some of that into into the modern financial, private and seeing how some of these topics translate. That's great, thank you. So for today's discussion, not going to be a surprise to anyone, I don't think we're going to be talking about machine learning and artificial intelligence. Neither one of these are really new concepts. The first introduction of artificial intelligence really goes back to the 1950s, early 1950s. And the ability to make this come to fruition was really grossly understated. I think they thought that within the decade, it would be pretty much developed and out there. And as everyone knows, you know, today, we're still working on it in different ways to leverage and enhance it. So parents, I hear a lot of times that machine learning and artificial intelligence, you know, they seem to be used interchangeably, correctly or incorrectly. Can you give us a little background on what machine learning is? And how does that really differ from artificial intelligence? Or how does it relate to artificial intelligence? At Chuck day, you wouldn't be surprised that this is a subject that comes up often, but in my time outdoors, but also before, and your usage of the word 1950s is actually a really good way to think about where the term artificial intelligence came from. It's the old Roper two terms for sure. If you go back to the 50s 60s, it was just when we were building medical equipment that could measure what the brain was doing, sometimes kind of, to almost a neuron level. So the idea was a new one receives a bunch of input along with dendrites into some kind of firing chamber, and then sometimes it will send a signal out, sometimes it wouldn't. And they realized back then that there was something called an activation threshold that will take place at the individual nerve was taking him on stage or doing something with that data. And then if a certain amount of energy was freed from that data, he would fire a message down the line to the next node. And just that simple, either adviser message, or it doesn't fire a message, it very quickly resonated with the computer scientists of the day who went well, if it's sending something or sending nothing, that's a one or zero, funnily enough, that's how all the computers that are currently working on work as well had it was around the same time that, you know, the people were revisiting some of the concepts that came from Alan Turing with the idea of one of the seminal papers, it can machines think. And it was a what if we know that a brain works with nerves, and we know that nerves, five zeros and ones, and we know computers by zeros and ones, I reckon we'll crack this or we just need as a lot of nerves, and we'll we'll simulate human brain, you know, within 20 years, everyone will have a robot companion. You know, we didn't see that clearly. But that's really where the term artificial intelligence came from. Could we replicate human level intelligence, in technology specifically, within software? The terms evolved, I would say over the last 25 years. Back when I was studying AI at university, it was very much focused on a kind of AI that was, can we replicate human intelligence? The majority of that is how do we represent the ways that we think humans are symbol processing simple generating machines. That kind of AI is is a little bit less sexy and enterprise right now. The kind of AI that was nice back then, but certainly is the big AI right now is machine learning, but the types of AI that sit on very different ends of this sector within the rise of machine learning very much lines up with the kinds of problems that big enterprise organizations trying to fix, and very specifically, that adaptive use case, if that makes sense within financial crime as well. Long after Ted, but hopefully that that helps shed some light on this. That was great. Thank you. Um, so you said that you are somewhat new to the financial crime area, you know, your background is really in artificial intelligence. When did you see or maybe this is a good question for you, since you're somewhat new, but when did you start seeing this get into the financial crime prevention space? So is a two part answer, it probably does know, but you don't get a short answer from me. And the answer 1999. And I can tell you that exactly, because my university project was to develop a basic neural network that could work out if someone was applying for a mortgage fraudulently or not. And back, then, it was very much a pattern classification engine, give me lots of examples of fortunate applications. And I will just figure out, if a new application is matching one of those or not, very, very basic neural network back then. It came as a surprise when I joined ACTA Mize three years ago, that you know, the stuff that I was doing, as someone who didn't know what he was doing, at the age of 20, I would have expected the world of finance to be the first adopter of these kinds of technologies and not only for me to walk into a world where it was in use, but it was leading the charge. Instead, if we look at what's happened with AI and machine learning particular is the market is that have taken advantage of over machine learning and have led the charge. You know, the the big companies, Google, Facebook, Amazon, probably developing some of the most interesting machine learning right now, that being said, if we look at financial crime, and certainly if I look at how my customers are using machine learning across all my different customers, so fraud is probably the biggest user of machine learning because it fits really well. That's it that where it kind of came into play was really in the fraud space before it started getting into AML and other areas within financial crime. Yeah, I think so I think that's because it fraud block near broadly speaking, trivializing an entire industry looks at two outcomes is something for or is something not for, give me a quick decision on that. That's exactly what your networks are for, you know, basic classifier model, what we'll do that very, very quickly, will run incredibly quickly will produce more accurate results, and you will expect of any human being when they create the wall space system. So Ford was definitely the the easiest entry point for, but as machine learning technologies have evolved, and you'll see greater degrees of explainability coming to machine learning, that now naturally lends itself better to things like money laundering, where it isn't just a case of is it? Yes or No, it's more complex complex than that, and the complexity required by the ability and and really, that's maybe why a lot hasn't been using it as much over the last 10 years, like fraud has that was absolutely seeing it now make more and more sense in in AML, as we can help bridge that gap between explainability and, and precision. So going on that theme I guess I am Why are we seeing it coming to the AML space, you know, if it we haven't seen it, you know recently? What is the purpose of it? Or how what's the Why is it coming into this area? predictable problems. It's because if you look at the landscape or what people are trying to do with transaction monitoring, if I pick that as my example in money laundering is a huge datasets massive, massive datasets, including billions and billions of people and organizations. You would think on paper that this is an area that you would certainly when you got large volumes of data movement patterns of data on paper, it's it's data scientists treatments, exactly the kind of problems that they look for. The challenge, I think has always been the impenetrability of or the the perception that machine learning was blackbox and impenetrable, unexplainable, and therefore, not applicable to the world of transaction monitoring to the world money laundering. But it's a it's a restatement or re articulation of the problem statement that is making people sit up and think, Oh, I get it now. You don't necessarily have to copy the fraud paradigm in money. Look, you don't have to build a model that says find me if something is money laundering or not. You can break the problem statement up. segmentation is an absolute perfect example of this. I speak to customers across the spectrum on a daily basis. Most of them will openly say they run somewhere between 12 and 15 population groups. So these are how did they group their customer base together. Businesses or businesses get grouped into hotels, if they're all hotels, they'll get booked Go to the restaurants or restaurants. And they know that the money laundering behavior that looking for is going to be different based on that type of business or someone else. But they limit themselves to how many business types that they can grip, how many segments or they can create. And that's simply because every time you add more business groupings in these different segments, you're increasing the amount of work a human has to do to try and tune a money laundering platform to find that exponentially sometimes, well, that's a great problem for a machine to solve. Because what you're saying is, I've got two different problems don't want I want to represent my customers better. unsupervised clustering, machine learning is absolutely perfect for them. off, we got suddenly we've got hundreds, there are groups that represent my customer better. But oh, no, now I've got a system that isn't optimized for hundreds. One of the problems that machine learning is very good at solving is the optimization problem. So absolutely directly now that we've got a new landscape to work with data data that's representing my customers better, let me give it to another machine to learn how to adapt itself to optimize itself for this new data set. And the result of which you suddenly start to introduce machine learning into that transaction monitoring paradigm, but not quite in the way that people initially thought they would want to, but it produces the kind of outcomes that they want to, and that just come from maturity of the data science space, and the maturity within the money laundering space to recognize it. Machine learning isn't a hammer that cracks every nut sometimes genius, you need to use machine learning in a different way. And the right way to figure out how to use it is figure out the problem you're trying to solve first, then apply machine learning. And I think if you go back 1015 years in the money laundering space, people were trying to apply the fraud paradigm to money laundering. And that's why it wasn't working, changing that paradigm changing the province, David has suddenly allowed people to recognize where it can be applied. Yeah, just going after that with you're talking about your segmentation. And putting people in or putting different clients into different peer groups, you know, going back 1015 years ago, it really was more, are you an individual? Or are you more of a corporate, and you have a rule set aside to both of them. But when you start looking at the activities between just an individual account student versus a high net wealth, individual, there's a lot of different risks that are involved in a lot of different transactional activities. And when you start to break it out into those different buckets to try and stop some of the money laundering and look for those anomalies, it gets very, very difficult to try and apply the rules for each one of those different groups. So I completely understand why this is very beneficial. And when you look at what we're doing, you know, in the financial space, we're doing a good job of capturing money laundering, but not good enough, you know, we're capturing less than 2% of it on a $2 trillion business every year. So yep, I see that we need to do something different than the way that we've been doing it. And this definitely is the right direction. With that being said, though, do you think this is something that's more hype in this area in something else is going to come along in the next five years? Or what do you see the next direction for this happening as it pertains to money laundering? Do I think it's hype? No. And I kind of have to say that not because I work at activists, but just because I was an ex core software developer who coded machine learning and neural networks for a long time before I moved into the solution role. And I believe it I this technology is absolutely viable. It absolutely works. The reason why I should have thought I should have said is it hype for the AML area. I'm not saying artificial intelligence hype. No, I believe that's here to stay. But yeah, I'm talking in the financial area. You know, you see a lot of different things that are happening that come along over time. And you believe that this is probably here to stay. Yeah, I did. Because it's always a problem. technologies that turn up and look great and actually solve a problem and end up tending to disappear. The Google Glasses a great example very smart, very machine learning driven technology, didn't solve a problem didn't actually do anything for anyone that they couldn't do by just holding their phone up. And in fact to introduce problems it creates a social awkwardness. Machine learning AI in the space of financial crime is solving genuine problems. So it's difficult to take something away when you know it's actually doing something useful. Do I think eventually, it will get replaced in its current form? Yes. Do I think it's five years away? No. And if we've got time happy to explain that, but whether I can ramble. I think this is probably a good time to take a short break. We will be right back. fraudster will always try to hide their true location to complies next level location intelligence. unmask bad Actors by utilizing location data to augment existing risk engines and fraud prevention solutions, increasing protection against account takeovers and by providing the industry's most accurate VPN and proxy detection technology. But to comply, fraud has no place to hide. To learn more, check out www dot geo comply.com. We're back with PR Patel, the head of solution consulting at nice ACTA Mize. So parent I've been on the other side of the table in financial institutions for over a decade. One thing that I saw that was very tricky was navigating our model governance and regulatory landscape. Machine learning add some in artificial intelligence adds some complications to this. Previously you're talking about it's looked at as a black box. Can you explain a little bit more on why they would model governance really is and why this causes a challenge. Yeah, it's the explainability is is a term that is often overused and misunderstood. And that often lies at the heart of what people think model governance is about model governments, depending on the area that you're speaking, really is saying in financial crime. How do I know the output of this machine learning process doesn't introduce new risk into my business, either from a fraud perspective or from a money laundering perspective, it doesn't matter just says, you've done something new. How do I know that new thing is not making my business more susceptible to a fraudster or is allowing money launderers to find an exploit and move money through my institution? So governance, model governance, particular as I say, Well, how do we evidence that? How do we build a framework that says, I don't want to give you data science facts, I don't want to give you an area under a curve and say, hey, look, the area under a curve, for my errors in a new model is better than the previous one. Because 99% of overt financial crime team would understand that they shouldn't have to data science produces funding, much of data science consumes a lot of data, data science produces a lot of data points, things that make sense to other data scientists. But these are not things that I would expect the head of compliance or head of fraud, to even want to understand they need to speak in the language of the problems that they have. And more governments is for me, that's what model governance is, how do we build a process that says in the context of money laundering in the context of transaction monitoring, if the machine is recommending that I make these changes, how can I evidence that these changes don't introduce new risk to my environment? And one of the ways that we can do that is we take this away from the sciences. And we say, Well, you've told me you've got something good, let me run it. But let me run it in a way that doesn't replace what I have already. Let me run it in a way that runs side by side and see against the same data. How does this new technology perform? How does this new model perform and build a report that makes sense to the head of transaction monitoring? Hey, before, you had 100, true positives and 50 false positives, now, you've still got 100 true positives, but you've only got 20 false positives. So you you're providing a framework in the language that speaks to the person who owns that problem. So that hopefully that answers that what you were asking that? It does. And I went through the process of doing like the first model validation, which, like you said, is really looking at all your inputs. What are you expecting? And what are your true outputs, it was a very cumbersome process. And that was on a rules based system many, many years ago, where it was very defined very prescriptive of this is what has to happen. And it was very difficult at that time, going forward with with something that's not quite as known and quite a straightforward. It does lend its complications. When we're looking at this from a regulatory standpoint. Do you think it's true there as well? Do you think that there's some issues on the regulatory landscape as far as explainability? and those types of things? Yeah. And then this goes into the, the, the explanation on what explainability? So if we break that statement down, from the perspective of certainly money laundering, what does expandability mean? If I was say, for example, building a topology that look restructuring lots of people or a person or a group of people transferring small amounts of money in a way that they think is going to bypass a money laundering detection technology then they are bleeding money through my system. It's an example of structuring, hopefully it's example structuring 10 you can always tell me if I'm, if I've got that technology wrong, but when you Tell the regulator's Hey look, I think I found something I think I've an instruction they want to know, not just that you found money or they're gonna want to know what kind of money laundering you found. If you applied the fraud way of thinking about detection into transaction monitoring, then all you're going to produce is something that says, hey, I found money laundering, we're not want tight. And so for a long time, money laundering, teens were hesitant to take advantage of machine and because they thought, well, all you're doing is shifting the explainability workload back onto my teams, your system is going to tell me Hey, I found some money laundering my team, they're going to have to go through to trawl through the transactions to figure out why the machine thinks money laundering took place, and they're gonna have to manually find that evidence of structure. That's no longer true nowadays, what you're seeing is that there's been a lot of research and development in the space of individual model expandability. So the machines can now not just say, Hey, I think I found a pattern, it will try and explain to you why what data is looked at that evidence is that it's found that so there is absolutely a movement to make that explainability a little bit more transparent. It's not quite there, you know, the science doesn't exist yet for fully explainable models. And there is always the, the balancing act of machine learning is, the more complex you make the machine learning models are bred to find more and more complex behavior types, the less explainable it is. But there is a happy balancing point that I think the industry is working together on to try and fight. And this may be where the regulators need to sharpen up their language a bit as well. We're seeing regulators say, to our customers, you should be using machine learning. That doesn't make sense. It's like going to someone who owns a car and said, you know, you should be using a spanner to do what we should be re articulating the usage of these kinds of technologies against a problem, not just thinking about as one big bit of software that you install. Machine learning is a process that it's a process that applies to a problem statement. But what I would like to see, as a regulator move away from say, you should use machine learning, dude, hey, bringing back segmentation, we think you can group your customers better. We'd like you to see, we'd like to see you apply some more machine learning techniques to do that. That makes more sense, because that's our problem state but that you can solve just say use machine learning to improve money laundering is too broad. and machine learning itself is not a piece of software, you can just get on a CD and install on your computer. It's very much process. No, I agree completely with that. But I am happy with the direction that the regulators are taking. Because as we had talked about, when this originally came out, it was more of this black box, people are a little bit afraid of it. So because of the explainability. But I've seen changes over time, you know, I see the FCA over in the UK is seems to be leading the pack with some of these new technologies they've done. Tech sprints and things like that. I think fincen kind of in the US seems to follow a little bit in the trends that they're doing. But now you're seeing a lot more encouragement. And I think it goes back to December of 2018, question mark, there was five organizations or regulatory bodies that actually were, were promoting the use of these newer technologies, which is great, because before they're apprehensive now that they're seeing the benefits of them, I think it's really good. And then the other thing that they said in that, from a financial perspective is that if you start to use some of these newer technologies, and you find things that you weren't finding before, we're not going to penalize you for that, because I think that a lot of financial institutions before, we're afraid that if we start finding new anomalies, we're gonna have to go and do look backs, and do all that kind of extra work, which they're a little bit afraid of, because that is a lot of work, if you've ever had to go through and to look back on, you know, of transactions over the last, you know, one to two years to try and find out what you might have been missing. So I think there's that apprehensive ness of the fact that they're going to give more of a safe harbor to those using this, I think was a good thing. And then the other piece of it was that they're not going to penalize organizations for not doing this, which I thought was just kind of an interesting thing. So the thought process that I had there was Why would you say that, and I'm wondering if it's, we're not going to penalize you now. But you might want to start thinking about this because it might be the new norm going forward. So with that, you know, we've already talked to this isn't a fad, this is an extra hype, you know, in the financial industry or areas. But where do you see this going in the next five years? Do you think this will become the new norm? Do you think that all financial institutions are going to have to do it? What about some of the smaller guys that don't have the resources? You know, where do you see this going? So I mean, it's going back to that point, I don't think it's going away. I do think your point is a good one. Should people have to do it? And no, I think it's gonna be very difficult to tell people that this is something that he must do. Is this something that people should do? Yes. A if I put my hippie hat on, I love the idea that we're Solving big world problems, you know, like you said 2 trillion in money laundering. This isn't your happy Chappie, you know, friendly drug dealer. These are human traffickers, you know, these people who are doing things that are detrimental to society? Do I think people should be using these technologies? Because it helps find more of these kinds of people don't use it? Yes. Do I think everyone can do it not because there's an economy of scale. The second, the mid to large organizations can take advantage of this because it is absolutely worth the investment. Because not only does it solve these real world problems, it solves the corporate problems that they may have it can reduce the workload within an organization while keeping their accuracy and precision quite high. Looking at the smaller organization, does it make sense for them to invest in a data science team? Just to focus on their problems? No. But that being said, that's one of the changes that I see. It's already happening now. But I've seen the trend of increasing of the expertise, how do we start to share data between different organizations so we can say, hey, look, you're a small organization, you don't have a data science team, you don't need a data science do. We've got shareable concept or shareable model machines that have learned from large data sets that we think are going to be applicable for you. So they can take advantage of the benefits of it without really needing to invest in their own full end to end data science program. So that, for me is what the next five years looks like. It's where it makes sense to people start to use more of these kinds of technologies. If I go beyond that, as I hinted at this earlier, the machine learning in its current form, eventually will reach that is kind of my opinion. But I seem to share that opinion with the head of google google brain, the guy based in London, it was a lot of investment on statistical machine learning, which are the current form that we're in. I personally think next 30 years, at some point, someone will crack the other side of that AI triangle. Which is that symbolic AI, which is how do you build a machine that understands the meaning of symbols that can generate meaningful symbols, you get to that point, suddenly, you're talking steps towards general intelligence, that's when you start to see that sci fi, ai start to become a reality. At that point, machine learning starts to become less viable, because there's no turning machine. Here is a year's worth of fraud day to go and learn how to detect fraud in the future, you actually say to them, she should give the definition of fraud. You know, this is what first party fraud is, you're now an employee of mine, go and stop it. And the machine will people will learn to pick it up. It's up. That's science fiction stuff. But that's what ultimately replaces machine and that we're on now. But he's decades away. It's decades away. In academic laboratories. It's even further away with the enterprise. I don't think we'll see it in our lifetime. But that that is what allegedly replaces this as a technology type. Well, we do have you recorded so we can go in 30 years and go back to this moment and see how close you were. Apparently, I'd like to thank you for joining me today. I hope that those of you listening found this content to be interesting and useful. Period. If people want to get in touch with you or learn more about your work, how can they do that? You can contact me at Peridot Patel at nice ACTA mize.com or I'm sure that if you drop us a line at the podcast at mosaic demo.com email address, your questions will certainly make their way across to me as well. Thank you, Perry. Thanks again for listening. And please don't forget to subscribe. If you have an idea for the show. We'd love to hear from you. Drop us a line at podcast at nice atomized calm. Don't forget we do have bonus content for every episode available at atomize. That nice.com slash podcast. I want to thank parrot again for being here with us and we'll see you on the next episode of Let's talk Vin crime.